Anonymizing, Pseudonymizing, Masking, or De-identifying?
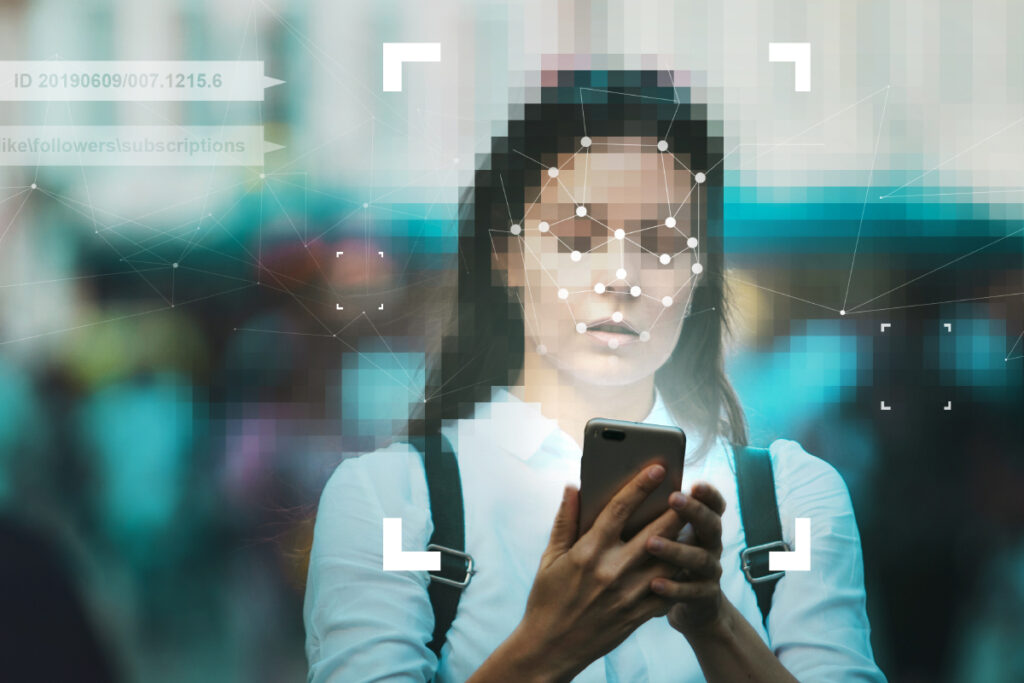
A variety of terms surface when discussing the protection of privacy-sensitive data. Many of these terms raise questions or lead to confusion about their precise meanings. It’s high time to shed light on these commonly used concepts!
Anonymizing
Let’s start with the term we use most frequently: anonymizing. Anonymizing personal data involves removing the connection between identifiable data and the natural person. Identifiable data refers to information that could potentially lead to the identification of the individual.
The General Data Protection Regulation (GDPR) provides the following definition for anonymous data: “data that do not relate to an identified or identifiable natural person.” [1]
Identifiable data falls into two categories: direct and indirect identifiable data.
Direct or Indirect Identifiable Data
Direct identifiable data allows you to identify a person based solely on that information. An example is the Social Security Number (SSN). Each person in the United States has a unique SSN. If you possess the SSN, you can use it to directly trace the person.
For indirect identifiable data, also known as quasi-identifiers, a combination of data is needed to identify a person. An example of indirect identifiable data is a zip code. Generally, multiple people live in the same postal code area, making it less likely to lead to the direct identification of a person. However, if we combine the zip code with another indirect identifiable data, such as the birthdate, there is a greater chance of identifying the person. For instance, how many people live in a postal code area with the birthdate January 17, 1953?
The more indirect identifiable data available, the higher the chance of identification. U.S. research has shown that 87% of Americans can be identified by combining just three indirect identifiable data points: zip code, gender, and birthdate [2].
Anonymous data have a significant advantage for organizations. If the data is anonymized to the extent that it can no longer be used (directly or indirectly) to identify a natural person, then these data no longer fall under the GDPR laws and regulations. In other words, this data can be used for all purposes without the need for additional measures.
Masking and De-identifying
Often, the goal of anonymizing is to enable the use of data for testing, development, and analysis purposes. Depending on the context in which the data is used, one must determine which data needs to be protected and in what way. It may well be possible that certain indirectly identifiable data are protected differently for one purpose than for another. For instance, in research, the requirements for a birthdate may only necessitate the birth year. In this case, a birthdate (14-09-1982) can be protected by changing it to just the year (1982) or by altering it to a random date within that year (such as 26-10-1982 or 03-05-1982).
The choices we make during anonymization determine the category within this overarching concept. Although we often use anonymizing as a general term, it encompasses two distinct categories: masking and de-identifying. Both anonymization categories aim to anonymize the data so that it is no longer traceable to individuals. However, the difference between the two categories lies in the usability of the data from an analytical perspective.
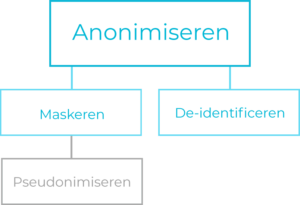
Anonymization methods falling under the masking category typically aim to thoroughly anonymize data without considering the usability of the data after anonymization. A simple example of this is replacing an email address with a predefined value such as XXXXXXXXXXX@XXXXXXX.XXX or completely removing the email address (also known as generalization). Through this masking, the data can no longer be used for analysis purposes. All data is the same, and nuances in the data disappear. Masking is often applied to directly identifiable data, such as the Social Security Number (SSN).
When anonymizing through de-identification methods, a different approach is employed. In essence, the goal is to ensure that the data remains usable for analysis purposes, while simultaneously making the data no longer traceable to an individual. An example of de-identification is adjusting the precision of the data, as described earlier in the example of the birthdate. Another method could involve dividing values in a dataset into categories. For instance, a person aged 33 falls into the category of 30 to 35-year-olds, while a person aged 18 falls into the category of 15 to 20-year-olds, and so on.
Directly identifiable data is often masked, while de-identification is commonly applied to indirectly identifiable data.
Pseudonymizing
So far, we’ve focused on the term anonymizing. In addition to anonymizing, pseudonymizing is often mentioned as a method to protect personal data. Anonymizing and pseudonymizing are closely related. Pseudonymizing is essentially a form of masking that falls under anonymizing; however, the purpose of both techniques is fundamentally different.
While anonymizing aims to protect data in a way that it can no longer be used to identify a person, pseudonymizing takes into account that the data can indeed be traceable through additional information. The GDPR provides the following definition of pseudonymizing: “processing personal data in such a way that the personal data can no longer be attributed to a specific data subject without the use of additional information…” [3].
Forms of pseudonymizing include encryption or replacing an existing value with another (unrelated) value, known as a pseudonym.
An example is replacing a Social Security Number (SSN) with a pseudonymous value. For instance, the SSN 149830751, a fictional number for illustration purposes, could be pseudonymized as 5A3GBN97Y. This renders the original SSN unrecognizable.
The strength of pseudonymizing lies in the uniqueness of each pseudonym for the original value. The aforementioned pseudonym 5A3GBN97Y is used only for the SSN 149830751. This allows linking data across different datasets since, in each dataset where the SSN 149830751 appears, it is replaced by the pseudonym 5A3GBN97Y. This wouldn’t be possible if we generated a random pseudonym for each unique value.
Another advantage of pseudonymizing is its reversibility. This means that the pseudonym can be translated back to the original value as long as you have the additional data that led to the pseudonym. This additional data could, for example, be an encryption key. If you have the correct encryption key, the pseudonym can be translated back to the original value. Without the key, there is no way to reverse the pseudonym.
Pseudonymizing is permitted according to the GDPR, as long as the additional data (such as the encryption key) is kept separate from the data, and sufficient technical and organizational measures are taken to protect the relevant additional data [3].
[1] “General Data Protection Regulation”. Article 4, recital 26.
[2] L. Sweeney, “Simple Demographics Often Identify People Uniquely”. Carnegie Mellon University, Data Privacy Working Paper 3.
[3] “General Data Protection Regulation”. Article 4, Definition 5.